background
Remote sensing has become versatile. There is neither one sensor nor one platform for all conceivable applications, but rather a large number of possible recording systems for every application. The sensors differ in the wavelength used (visible light, infrared radiation, microwaves), in the lighting (active or passive) and in the recording geometry (point cloud, central projection, parallel projection, distance projection). The selection of platforms ranges from the fixed camera to unmanned aerial vehicles (UAV), airplanes and helicopters to satellites and space stations.
The two basic differences resulting from the choice of platform are the distance to the observed object and the repetition rate, i.e. the time interval at which exactly the same recording can be repeated in order to detect changes. If you want to use this broad spectrum of data in all its abundance, you need advanced approaches for data fusion and automatic interpretation.
Methods of machine learning have established themselves for this – not only in remote sensing – but in turn require extremely large training data sets in order to learn the syntactic patterns and link them to their semantic meaning. Such training data sets are still scarce in the field of remote sensing, since the reference data for the clear assignment of a semantic in the form of so-called labels are usually missing. Procuring them is extremely time-consuming and expensive and exceeds the usual budget for a remote sensing project.
Goals
The "Wald5Dplus" project, funded by the space management of the German Aerospace Center e.V. with funds from the Federal Ministry for Economic Affairs and Energy, creates a syntactic training data set from multitemporal Sentinel-1 and Sentinel-2 satellite images and assigns the individual elements semantic labels that derived from flights. The satellite missions Sentinel-1 (C-Band Synthetic Aperture Radar) and Sentinel-2 (Multispectral Imager) are part of the Copernicus program of the European Commission and the European Space Agency. They provide weekly recordings in a 10 m grid of the whole of Europe (unfortunately depending on the weather in the case of Sentinel-2), which are freely available to every potential user. In a year, around sixty images are collected per satellite. As part of this project, the grid values are calculated for three selected forest areas in the north-south direction (first dimension), in the east-west direction (second dimension), polarimetrically by Sentinel-1 (third dimension), spectrally by Sentinel-2 (fourth dimension ) over time (fifth dimension) in an Analysis Ready Data Cube and provided with semantic labels (“plus”). The labels come from flights of the test areas with aircraft or UAV-borne laser scanners and multispectral cameras and therefore have a very high spatial resolution. They are intended to provide information about certain forest parameters such as tree species, number of trees, crown area, crown height and initial crown height. The point clouds are evaluated using specially developed, patented algorithms and result in the required semantic labels, which are then aggregated onto the spatial grid of the satellite data.
Using the data cube, machine learning methods are pre-trained in order to be used in other projects. The entire package will be made available to all interested scientists worldwide free of charge as a benchmark data set via the CODE-DE platform.
Results so far
At the end of the project period, analysis-ready data cubes with weekly recordings of Sentinel-1 and Sentinel-2 over a period of two years will be available for three study areas in the Bavarian Forest National Park, in the Landesaboretum "Weltwald Freising" and in the Steigerwald. To reduce storage space and allow for easier interpretation, the recordings are polarimetrically, spectrally and temporally fused on hypercomplex bases. In addition, each image element is assigned a typical forest parameter such as the number of trees, the proportion of deciduous or coniferous forest, the average canopy height, etc.
This data set is analyzed in detail using methods of multivariate statistics and machine learning in order to optimize the data preparation on the one hand and the transfer of what has been learned to other areas on the other. All results are also published in international specialist journals with open access. From now on, the data set, including pre-trained algorithms, will serve as a benchmark against which new developments in the field of artificial intelligence can be measured. In the optimal case, a sufficiently validated AI algorithm can be offered that can estimate the forest parameters introduced based solely on images from the Sentinel-1 and Sentinel-2 missions.
outlook
With the help of this benchmark data set, AI algorithms can be classified consistently in the future. They all train on the same Analysis Ready Data Cube with the same labels and predict the forest parameters for the same syntactic signatures. Then only the accuracy of the assignment and the performance of the algorithm decide on the respective list position.
In this way, the state of the art can be objectively evaluated for the first time. For example, one could select the optimal algorithm from the list of possible candidates in order to carry out a thematically detailed forest mapping across the board based on the freely accessible data from Sentinel-1 and Sentinel-2. Unexpected changes such as forest damage caused by diseases, pest infestations or storms could also be detected promptly so that countermeasures can be taken in good time to prevent further spread and thus greater financial damage.
Methodologically, the benchmark data set offers the optimal starting point both for the validation of the already published algorithms for data fusion on hypercomplex bases and for thematic interpretation via histogram classification as well as for the development of new or adaptation of existing machine learning algorithms for use in forestry remote sensing and far beyond.
grantor
Bundesministerium für Wirtschaft und Klimaschutz
publications
Schmitt, A., Wendleder, A., Kleynmans, R., Hell, M., Roth, A., Hinz, S., Multi-Source and Multi-Temporal Image Fusion on Hypercomplex Bases. Remote Sens. 2020, 12, 943. (https://www.mdpi.com/2072-4292/12/6/943)
Krzystek, P., Serebryanyk A., Schnörr, Cl., Cervenka, J., Heurich, M., Large-scale mapping of tree species and dead trees in Šumava National Park and Bavarian Forest National Park using lidar and multispectral imagery. Remote Sensing 2020, 12(4), 66.1. (https://doi.org/10.3390/rs12040661)
Schmitt, A., Sieg, T., Wurm, M., Taubenböck, H. , Investigation on the separability of slums by multi-aspect TerraSAR-X dual-co-polarized high resolution spotlight images based on the multi-scale evaluation of local distributions, International Journal of Applied Earth Observation and Geoinformation, Volume 64, February 2018, Pages 181-198, ISSN 0303-2434. (https://doi.org/10.1016/j.jag.2017.09.006)
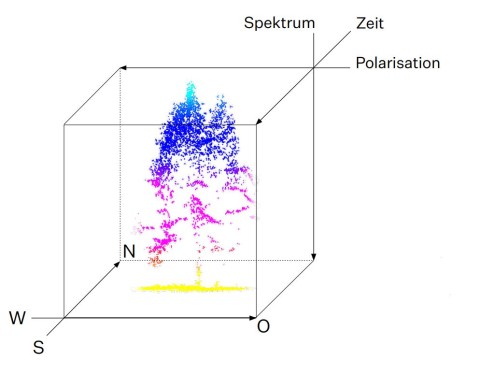