Ampel
Knowledge discovery methods and automatic model generation for multichannel marketing
Motivation:
Social networks such as Facebook, Twitter, Instagram & Co. are now used by almost all companies in marketing. However, this sometimes incurs high costs. The question arises, which tools can be given to a company to determine a prediction for the benefit of social media investments.
Objective of the project:
The objective of the research was to explore how social media (SM) channels work for businesses. A solution was to be found that would support demonstrating a corresponding benefit effect when using a limited budget. A knowledge discovery method should be developed that automatically detects the impact chains in multiple SM channels and displays them.
Procedure:
New metrics were defined to measure the success of a contribution. In the first step, data from the automotive sector (BMW, Daimler-Benz, VW, Audi) was collected - only later were additional data sets added.
In the course of the project, training data was collected as well as annotated and machine learning algorithms were tested and evaluated. The collection of Facebook data was realized with the help of the Facebook Graph API. This API is a web interface implemented according to the REST standard, which makes Facebook's data available in a structured form. The data collection was extended by an algorithm for further collection. The viewed pages are retrieved at regular intervals to collect new posts and user interactions.
In the project, the decision was made to first elaborate the approach based on Facebook and evaluate it with the help of a proof-of-concept, and then integrate the other SM channels in a further step. A generic data model was developed in which data from different SM channels can be stored in a uniform manner.
For the sentiment analysis, the collected dataset was cleaned and split to sentence level. The 6,000 sentences in the dataset were classified by sentiment into three levels: positive, neutral, and negative. Neural networks (Convolutional and Recurrent) were used as adaptive mechanisms. Some necessary data additions had to be done manually and could not be added automatically. These included the assignment to a category and the evaluation of success. As part of the experts' creation of the gold standard, each of the approximately 6,000 contributions was assigned to one of eleven categories. A first model for the automated categorization of new contributions, was tested on the basis of Support Vector Machine (SVM) and Logistic Regression, whereby a correct assignment to the categories successfully and unsuccessfully of approx. 30% (Support Vector Machine), respectively 15% (Logistic Regression) could be achieved. The low rate of correctly assigned contributions is due to the partly different assessment of the categories by the experts. In order to allow the clearest possible assignment of the contributions to individual categories, the categories were subsequently revised. In the further course, it was determined that the category had no significant influence on the success of a contribution. Therefore, further development of this model was discarded.
Based on the draft goal statement, a prototype tool was implemented. This enables the user to receive an assessment of the potential success of a new contribution while it is still being drafted, even before the contribution is published. The prognosis can take on three values, ranging from unsuccessful to partially successful to successful. The result is represented by a traffic light, which can assume the colors red (unsuccessful), yellow (partially successful) and green (successful). The findings from the deviations between the forecast and the evaluation can then be used to optimize the model for the forecast.
Two models were formed for the evaluation of success, differing in the timing of the evaluation. The first model allows to forecast the success of a contribution even before its publication, i.e., a priori, whereas the second model evaluates the actual success of a published contribution retrospectively, i.e., a posteriori.
The models show a very high quality in the evaluation of success.
Funding code:
IUK482/001
Cooperation partner:
ALTHALLER communication Gesellschaft für Marktkommunikation
Project funding:
IuK Bavaria
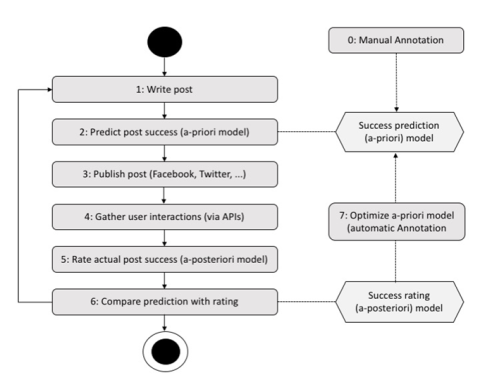