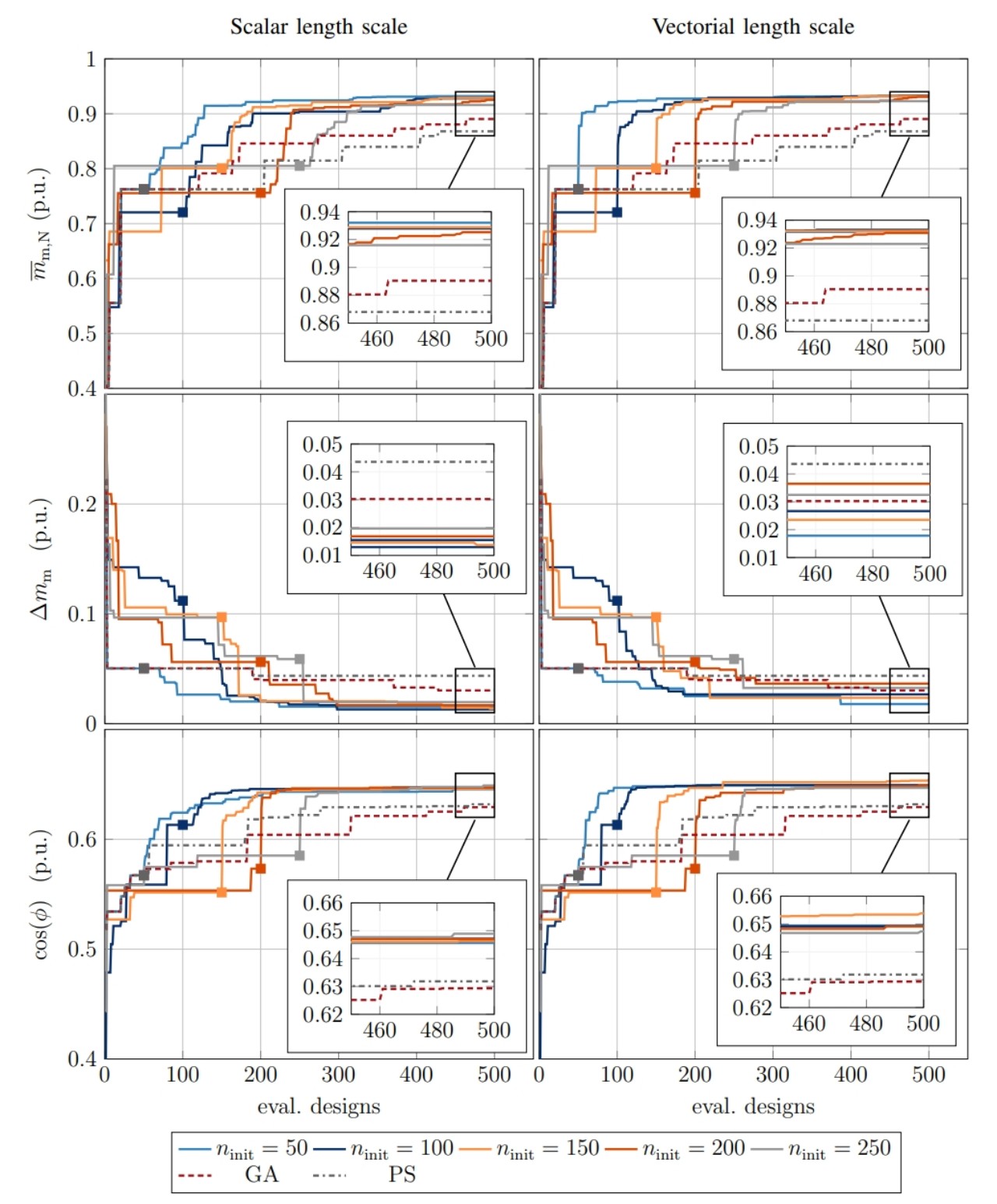
Single-Objective Machine Design with Gaussian Process Regression and Bayesian Optimization
Due to the complex rotor design of reluctance synchronous machines, a finite element analysis is essential for the accurate calculation of machine relevant performance objectives. Reluctance synchronous machines tend to have a large torque ripple, if this objective is not considered during the machine design. This necessitates a large number of simulation steps, resulting in a high computational burden and a long simulation time per design evaluation. Therefore, an efficient optimization algorithm is required. This paper proposes a complete framework for single-objective machine design optimization using Gaussian process regression and Bayesian optimization. Focusing on reluctance synchronous machine design, different kernel functions (squared exponential, Matern, rational quadratic) and hyperparameter configurations are assessed for regression accuracy of the optimization objectives mean torque, torque ripple, and power factor. The impact of noise in the input data on the regression results is also investigated. Bayesian optimization with the infill criterion Expected Improvement is finally used to perform machine design optimization for 18 design variables. Bayesian optimization outperforms the classical algorithms such as genetic or particle swarm algorithms. It results in a faster design optimization, even for such a high number of design variables.
Further information can be found here.