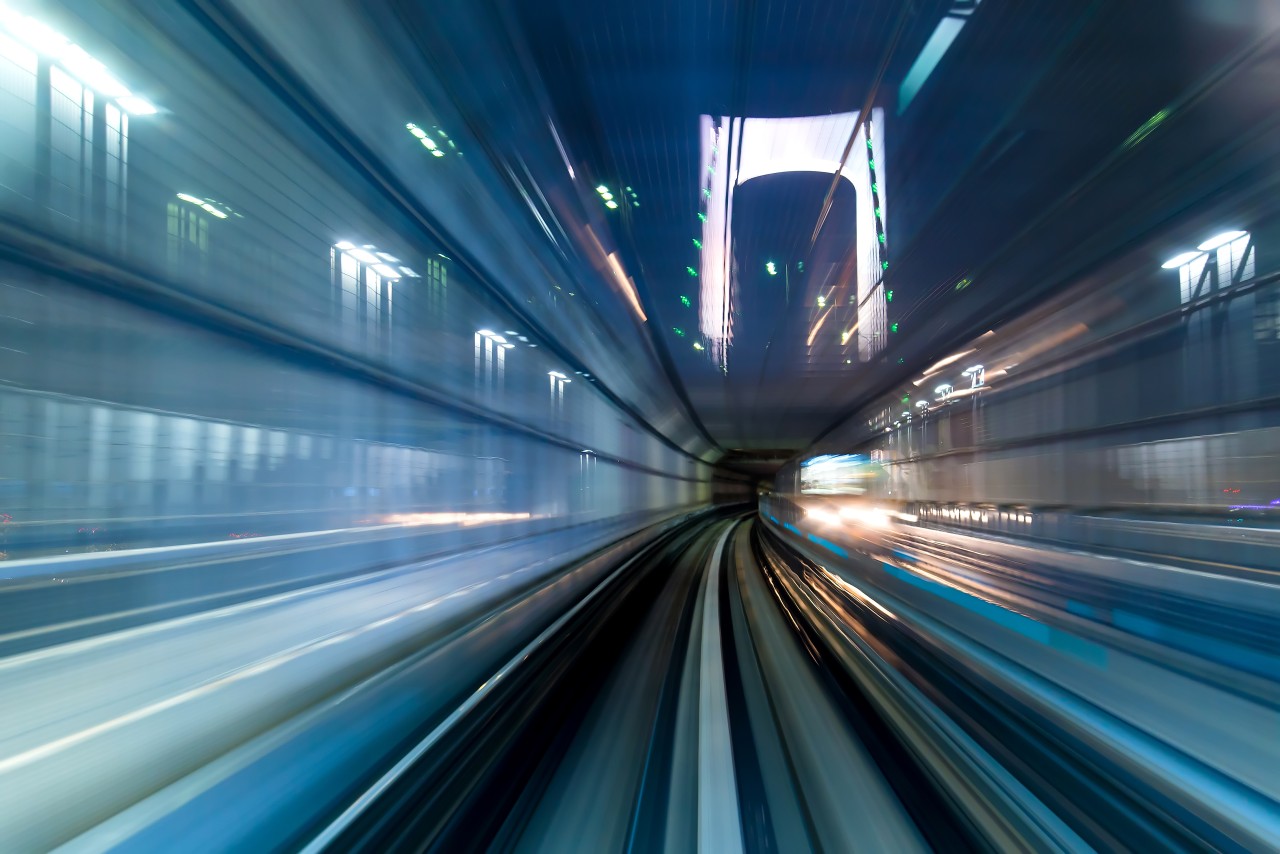
Multiobjective Design Optimization of Hybrid Electric Rail Vehicle Powertrains
Upsetting the technologies for rail vehicle propulsion systems
While existing electrification approaches fail to meet cost targets for many application scenarios, established cost-efficient diesel vehicles start to fall victim to CO 2 regulations. Innovative hybrid electric powertrain technologies have the potential to stop this predicament but suffer from low degrees of engineering experience. Within this VUCA (volatile, uncertain, complex, and ambiguous) environment, the paper on hand reduces railway powertrain decision making risks by applying the Hyper Space Exploration (HSE) approach to quantity the potential of innovative hybrid diesel-electric alternatives during design phase. Thereby stated multicriterial Pareto-optimal target trade-offs are identified by applying multiobjective optimization (MOO) algorithms on virtual prototypes. As underlying simulations with the Toolbox for Optimal Railway Propulsion Architectures (TORPA) are computationally expensive, efficiency of MOO search algorithms is of crucial importance.
Accordingly, three types of search algorithms are compared concerning their performance: Latin Hypercube Sampling (LHS), a Genetic Algorithm (NSGA2), and the Surrogate optimization of Computationally Expensive Multiobjective Problems (SOCEMO) approach. The outcome of this work enables railway product managers, system architects, and operators to choose appropriate search algorithms for effectively and efficiently quantifying the potentials of innovative rail vehicle powertrain technologies and, thereby, reduce their risk of decision making.
Read more details in the published article.